Arora–Rao–Vazirani Approximation for Expansion
In this lecture, we consider the problem of finding a set with smallest possible expansionIn the context of this algorithm, the computational problem being solved is typically called Sparsest Cut. We will refer to this problem as Min Expansion in order to be consistent with earlier lectures. in a given graph. Earlier we saw that degree-2 pseudo-distributions allow us to achieve non-trivial approximation guarantee, which turns out to be closely related to Cheeger’s inequality from spectral graph theory (see lecture 2). We also showed that this guarantee is tight for degree-2 pseudo-distributions (see lecture 3). In this lecture, we investigate the question if higher-degree pseudo-distributions allow us to achieve better approximation guarantee. An indication that higher degree might indeed help is that certain linear programming approaches, that are captured by higher-degree pseudo-distributions, achieve approximation guarantees for expansion that are better than those from Cheeger’s inequality in some range of parameters (Leighton and Rao 1988) . (In particular, those linear programming approaches certify tight lower bounds on the expansion of cycle graphs, for which no good degree-2 sos certificates exist.)
We will show that degree-4 pseudo-distributions achieve approximation guarantees for expansion (Arora, Rao, and Vazirani 2004) that for a large range of parameters are significantly stronger than both the guarantees of degree-2 pseudo-distributions and the guarantees of previous linear programming approaches.
Our exposition (especially the proof of the “structure theorem”) differs significantly from others in the literature. However, it borrows heavily from lectures notes of Rothvoss (2016).
Pseudo-distributions for expansion
Let \(G\) be a \(d\)-regular graph with vertex set \([n]\).The treatment of expansion here also works for non-regular graph. We assume regularity in order to be consistent with earlier definitions in the context of Cheeger’s inequality and degree-2 sum-of-squares. Let \(f_G\from \bits\to\R\) be the function that outputs for every vector \(x\in\bits^n\) the number of edges \(f_G(x)\) cut by \(x\) (viewed as a bipartition of the vertex set), \[ f_G(x)= \sum_{\set{i,j}\in E(G)} (x_i-x_j)^2\,. \] We identify the vector \(x\in \bits^n\) with the subset \(S=\{i\in[n]\mid x_i=1\}\) indicated by \(x\). For every \(i,j\in [n]\), the function \((x_i-x_j)^2\) indicates whether \(i\) and \(j\) are on different sides of the bipartition corresponding to \(x\). Recall the expansion of \(G\), \[ {\varphi}(G) = \min_{x\in \bits^n} \frac{f_G(x)}{\tfrac d n \card{x}\cdot (n-\card{x})}\,. \label{eq:expansion} \] Here, \(\card{x}=\sum_{i=1}^n x_i\) is the size of the set indicated by \(x\).
Note that actual probability distributions \(\mu\from \bits^n\to \R_{\ge 0}\) satisfy \(\E_\mu f_G \ge {\varphi}(G)\cdot \E_{\mu(x)} \frac d n \cdot \card x (n-\card x)\) and equality is achieved by distributions supported on sets with minimum expansion. If we consider degree-4 pseudo-distributions, we can find in polynomial time one such that the ratio of \(\pE_\mu f_G\) and \(\pE_{\mu(x)}\tfrac d n\card{x}(n-\card x)\) is as small as possible—certainly no more than \({\varphi}(G)\). The following theorem shows that this ratio can never be much smaller than \({\varphi}(G)\) and that we can extract from the pseudo-distribution a set with small expansion at most \({\varphi}(G)\cdot O(\sqrt {\log n})\).
Let \(G\) be a \(d\)-regular graph with vertex set \([n]\) and let \(\mu\from \bits^n\to \R\) be a degree-\(4\) pseudo-distribution. Then, \[ {\varphi}(G)\le O\Paren{\sqrt{\log n}} \cdot \frac{\pE_{\mu} f_G} {\pE_{\mu(x)}\tfrac d n \card{x}\cdot (n-\card{x})}\,. \label{eq:relaxation} \] Furthermore, there exists a polynomial-time algorithm that given \(G\) and \(\mu\) finds a vector \(x\in \bits^n\) witnessing this bound on \({\varphi}(G)\).
A birds-eye view of the proof
The proof of Reference:arora-rao-vazirani-approximation-min-expansion consists of several part. We describe each of them at a high level and explain how they fit together.
The first component is an algorithm called region growing. Given two subsets \(A,B\subseteq[n]\) this algorithm tries to find the best cut of the graph between \(A\) and \(B\) guided by the pseudo-distribution \(\mu\). As we will see, this algorithm achieves a \(O(1/\Delta)\)-approximation (compared to the pseudo-distribution) if the sets \(A,B\) are \(\Delta\)-separated in the sense that \(\card{A},\card{B}\ge \Omega(n)\) and \(\E_{\mu(x)} (x_i-x_j)^2\ge \Delta\) for every \(i\in A\) and \(j\in B\).
The second component is a structure theorem for pseudo-distributions that shows that for some \(\Delta\ge \Omega(1)/\sqrt{\log n}\) such \(\Delta\)-separated sets always exist.The actual proof requires a small case distinction here which we are going to ignore for this high-level discussion.
To prove this structure theorem we apply the quadratic sampling lemma (from lecture 1) in order to obtain Gaussian variables \(X_1,\ldots,X_n\) with the same first two moments as the pseudo-distribution. A natural first attempt to construct separated sets from those variables is to choose \(A\) to be all vertices \(i\in [n]\) such that \(X_i\) is significantly below its mean, say \(X_i \le \E X_i -1\) and to choose \(B\) to be all vertices \(j\in[n]\) such that \(X_j\) is significant above its mean, say \(X_j\ge \E X_j +1\). Indeed, this construction ensures that \(A\) and \(B\) are \(\Delta\)-separated for some \(\Delta\ge \Omega(1)/\log n\) (which in turn gives an \(O(\log n)\) approximation).
To improve over this first attempt, we post-process the sets \(A\) and \(B\) by iteratively removing pairs \(i\in A\) and \(j\in B\) that violate the separation condition, i.e., \(\E (X_i-X_j)^2\le \Delta\). The heart of the proof is to upper bound the number of disjoint pairs of this form. We will show that its expectation is at most \(O(n)\cdot (\Delta \cdot \sqrt{\log n})^{\Omega(1)}\). It follows that if we choose \(\Delta=c\sqrt{\log n}\) for a sufficiently small constant \(c>0\), then only a small fraction of vertices are removed in the post-processing phase in expectation.
To upper bound their expected number, we relate the above disjoint pairs to maxima of Gaussian processes defined in terms of the variables \(X_1,\ldots,X_n\). A crucial ingredient is an upper bound on the variance (and concentration) of the maximum of a Gaussian process and the fact that the Gaussian variables \(X_1,\ldots,X_n\) satisfy squared triangle inequalities \(\E (X_i-X_j)^2\le \E (X_i-X_k)^2 + \E (X_k-X_j)^2\) (because the pseudo-distribution \(\mu\) satisfies this inequality and \(X_1,\ldots,X_n\) have the same first two moments as \(\mu\)).
Region growing
Let \(G\) be a \(d\)-regular graph with vertex set \([n]\). Let \(\mu\from \bits^n\to \R\) be a degree-\(4\) pseudo-distribution. Let \(d(i,j)=\pE_{\mu(x)}(x_i-x_j)^2\) be the probability under \(\mu\) that \(i\) and \(j\) are on different sides of the bipartition. For a subset \(S\subseteq [n]\), we let \(d(i,S)=\min_{s \in S}d(i,s)\). Since the inequality \((x_i-x_j)^2 + (x_j-x_k)^2 \ge (x_i-x_k)^2\) holds over the hypercube for all \(i,j,k\in[n]\) and this inequality as a deg-4 sos proof, we have the following triangle inequality for all \(i,j,k\in [n]\) \[ \label{eq:squared-triangle-inequality} d(i,j) + d(j,k) \ge d(i,k)\,. \]
The following lemma is the main tool for extracting a set with small expansion out of a degree-4 pseudo-distribution.
Suppose for \(\Delta>0\) there exists a subset \(A\subseteq [n]\) such that \(\card {A}\cdot \sum_{i=1}^n d(i,A) \ge \Delta \cdot \sum_{i,j=1}^n d(i,j)\). Then, \[ {\varphi}(G)\le 1/\Delta \cdot \frac{\pE_{\mu}f_G}{\pE_{\mu(x)}\tfrac dn \card x (n-\card x)}\,. \].
Furthermore, one of the sets \(A_t=\set{i\in [n] \mid d(i,A)\le t}\) for \(t\ge 0\) witnesses this bound on the expansion.
Consider the following distribution \(\mu'\) over the hypercube:
- choose \(t\) uniformly at random in the interval \([0,1]\)
- output the vector \(x'\in \bits^n\) with \(x'_i=1\) if and only if \(d(i,A)\le t\).
Then, every pair \(i,j\in[n]\) with \(d(i,A)\le d(j,A)\) satisfies \[ \begin{aligned} \E_{\mu'(x')} (x'_i-x'_j)^2 & = \Pr_{t\in [0,1]}\Set{ d(i,A) \le t \le d(j,A) } \\ & = \abs{d(i,A)-d(j,A)} \end{aligned} \] By the triangle inequality \eqref{eq:squared-triangle-inequality}, \(d(i,A)-d(j,A)\le d(i,j)\). Therefore, \(\E_{\mu'} f_G\le \pE_{\mu} f_G\).
At the same time, since \(\card{x'}\ge \card{A}\) and \(\Pr_{\mu'(x)}\Set{x'_i=0}=\Pr_{t\in [0,1]}\Set{t<d(i,A)}=d(i,A)\), \[ \begin{aligned} \E_{\mu'(x')} \card{x'}(n-\card{x'}) & \ge \card{A} \cdot \sum_{i=1}^n d(i,A) \\ & \ge \Delta \cdot \sum_{i,j\in [n]} d(i,j) \\ & = \Delta \cdot \pE_{\mu(x)} \card{x}\cdot (n-\card{x})\,. \end{aligned} \] It follows that the expansion of \(G\) satisfies \[ {\varphi}(G)\le \frac {\E_{\mu'} f_G}{\E_{\mu'(x')} \card{x'} (n-\card {x'})} \le \frac 1 \Delta \cdot \frac {\pE_{\mu} f_G}{\pE_{\mu(x)} \card{x} (n-\card {x})} \,. \] Furthermore, there exists a vector \(x'\in \bits^n\) in the support of \(\mu'\) that witnesses this bound on the expansion.
It remains to show that we can always find a subset \(A\) as above for \(\Delta\ge \Omega(1/\sqrt{\log n})\). For simplicity, we first focus on the following special case,If the graph has a sparsest cut such that the smaller side has size at least \(0.2n\), we can require this inequality for the pseudo-distribution without loss of generality. Furthermore, it turns out that even the general case can be reduced to this special case. We present this reduction at the end of these notes. \[ \sum_{i,j\in [n]} d(i,j) \ge 0.1 \cdot n^2\,. \label{eq:well-spread} \] In this special case, we show that for some \(\Delta \ge \Omega(1/\sqrt{\log n})\) there exist sets \(A,B\subseteq [n]\) with \(\card{A},\card{B}\ge \Omega(n)\) such that \(d(i,j)\ge \Delta\) for all \(i\in A\) and \(j\in B\). Indeed for this choice of \(A\), the condition of the region growing lemma is satisfied, \[ \card{A} \cdot \sum_{i=1}^n d(i,A) \ge \card{A} \cdot \card{B}\cdot \Delta \ge \Omega(\Delta \cdot n^2) \ge \Omega(\Delta) \cdot \sum_{i,j\in[n]} d(i,j)\,. \] By Reference:region-growing, we obtain a \(O(1/\Delta)\le O(\sqrt{\log n})\)-approximation for the expansion of \(G\).
Structure theorem
The quadratic sampling lemma allows us to translate the task of finding sets \(A\) and \(B\) as above to a question about \(n\) jointly-distributed Gaussian variables. Without loss of generality we may assume that \(\E_{\mu(x)} x = \tfrac 12 \cdot \Ind\).The definition of expansion \eqref{eq:expansion} is symmetric in the sense that both \(x\) and its complement \(\Ind-x\) have the same value. Therefore, we can symmetrize the pseudo-distribution in the same way as for Max Cut.
Let \(X=(X_1,\ldots,X_n)\) be a Gaussian vector that matches the first two moments of \(x-\tfrac 12 \cdot \Ind\) under the pseudo-distribution \(\mu\). Then, \(X\) satisfies \(\E X_i=0\), \(\E X_i^2\le 1\), and \(\E (X_i-X_j)^2=d(i,j)\) for all \(i,j\in [n]\).
The following theorem about such Gaussian vectors shows that sets \(A\) and \(B\) as above exist and that we can find them efficiently. Together with Reference:region-growing this theorem implies Reference:arora-rao-vazirani-approximation-min-expansion in the special case that the pseudo-distribution satisfies \eqref{eq:well-spread}
Let \(X=(X_1,\ldots,X_n)\) be a centered Gaussian vector with \(\E X_i^2\le 1\) for all \(i\in[n]\). Suppose \(d(i,j)=\E (X_i-X_j)^2\) satisfies the inequalities \eqref{eq:squared-triangle-inequality} and \eqref{eq:well-spread}. Then, there exists sets \(A,B\subseteq [n]\) with \(\card{A},\card{B}\ge \Omega(n)\) such that \[ \min_{i\in A ,j\in B} d(i,j) \ge \Omega\left(1/\sqrt{\log n}\right)\,. \] Furthermore, we can find such sets \(A\) and \(B\) in polynomial time.
It will useful to rephrase the above theorem in terms of vertex separators in certain graphs associated with the Gaussian vector \(X\). For \(\Delta>0\), let \(H\) be the directed graph with vertex set \([n]\) such that \[ E(H) = \{ (i,j)\in [n]^2 \mid d(i,j) \le \Delta\}\,. \label{eq:geometric-graph} \] We say that subsets \(A,B\subseteq [n]\) form a vertex separator if no edge of \(H\) goes directly between \(A\) and \(B\), i.e., \(E(H)\cap A\times B=\emptyset\). We say such a vertex separator is good if \(\card A \cdot \card B \ge \Omega(n^2)\). With this terminology in place, we see that in order to prove Reference:structure it is enough to show that there exists \(\Delta\ge \Omega\Paren{1/\sqrt {\log n}}\) such that \(H\) has a good vertex separator.
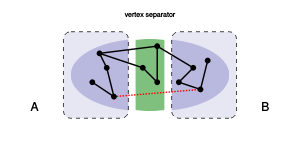
Proof of structure theorem
Let \(X=(X_1,\ldots,X_n)\) be a centered Gaussian vector with \(\E X_i^2\le 1\) for all \(i\in[n]\). Let \(d(i,j)=\E(X_i-X_j)^2\) for all \(i,j\in [n]\). Suppose that \(d\) satisfies \eqref{eq:squared-triangle-inequality} and \eqref{eq:well-spread}. For \(\Delta>0\), let \(H\) be the graph with vertex set \([n]\) and edge set \eqref{eq:geometric-graph}.
We consider the following randomized algorithm to find vertex separators for \(H\):
- Choose subsets \(A^0,B^0\subseteq [n]\) such that \[ A^0 = \{ i \mid X_i \le -1\} \text{ and } B^0 = \{ j \mid X_j \ge 1\}\,. \]
- Find a maximal matching \(M\) in the edges \(E(H) \cap A^0 \times B^0\).
- Output the sets \(A=A^0 \setminus V(M)\) and \(B=B^0 \setminus V(M)\).
Note that the objects constructed by the algorithm, in particular the sets \(A\) and \(B\) and the matching \(M\), are jointly distributed with the Gaussian vector \(X\). We emphasize that the edges of the matching \(M\) are directed. In particular, the event \((i,j)\in E\) implies that \(X_j-X_i\ge 2\).
We observe that the output \((A,B)\) of the algorithm forms a vertex separator in \(H\). Indeed, by the maximality of \(M\), every edge \((i,j)\in E(H)\cap A^0 \times B^0\) has at least one endpoint in \(V(M)\), which means that \((i,j)\) is not contained in \(A\times B\).
We also observe that if we find the maximal matching in step 2 by greedily passing over the edges in a canonical order independent of the randomness of \(X\), then for every \(x\in \R^n\), the matching produced in the event \(X=x\) is the reverse of the matching produced in the event \(X=-x\). It follows that for every vertex \(i\in [n]\), \[ \Pr\{ \text{$i$ has outgoing edge in $M$}\} = \Pr\{ \text{$i$ has incoming edge in $M$}\} \,. \]
The following lemma characterizes the success of the above algorithm in terms of the expected size of \(M\).
Either the above algorithm outputs a good vertex separator for the graph \(H\) with probability \(\Omega(1)\), or the random matching \(M\) produced by the algorithm satisfies \(\E \card {M}\ge \Omega(n)\).
A similar analysis as in the Goemans–Williamson algorithm for Max Cut shows that there exists an absolute constant \(c>0\) such that \(\Pr\{ X_i\le -1 \text{ and } X_j \ge 1\}\ge c\cdot d(i,j)\) for every \(i,j\in [n]\). Thus, by inequality \eqref{eq:well-spread}, \[ \E \card{A^0} \cdot \card{B^0} \ge 0.1 c \cdot n^2\,. \] Therefore, \[ \E \card{A}\cdot \card{B} \ge \E \card{A^0} \cdot \card{B^0} - n \cdot \E \card M \ge 0.01 c \cdot n^2 - n \cdot \E \card M\,. \] It follows that \(\E \card M\ge \tfrac 12 \cdot 0.1 c \cdot \E \card M\). (Otherwise, \(\E \card{A}\cdot \card{B}\ge \Omega(n^2)\), which would means that the algorithm outputs a good separator for \(H\) with probability \(\Omega(1)\), contradicting the premise of the lemma.)
We will analyze the expected size of the random matching \(M\) by relating it to the maximum of a Gaussian process.We remark that the lemma only uses the triangle inequalities \eqref{eq:squared-triangle-inequality} but not inequality \eqref{eq:well-spread}.
The expected maximum of the Gaussian process \(\{X_j-X_i \mid i,j\in [n]\}\) satisfies \[ \frac {\Omega(1)} \Delta \cdot \Paren{\E \card M /n }^3 \le \E \max_{i,j\in [n]} X_j - X_i \le \sqrt{2\log n}\,. \]
Together Reference:vertex-separator-or-large-matching and Reference:maximum-of-gaussian-process allow us to prove Reference:structure.
We prove the contrapositive: if the above algorithm fails to find a good vertex separator in \(H\), then the distance parameter \(\Delta\) of the graph satisfies \(\Delta \ge \Omega(1/\sqrt {\log n})\).That means that if we choose \(\Delta\) a sufficiently small constant factor times \(1/\sqrt {\log n}\), the algorithm will succeed in finding a vertex separator for the corresponding graph \(H\). Let \(M\) be the random matching defined by the algorithm. Since the algorithm fails to find a good vertex separator Reference:vertex-separator-or-large-matching gives a lower bound on the expected size of the matching, \(\E \card {M}/n\ge \Omega(1)\). On the other hand, Reference:maximum-of-gaussian-process allows us to upper bound the same quantity \(\E\card {M}/n \le O\paren{\Delta \sqrt{\log n}}^{1/3}\). We conclude \(\Delta \cdot \sqrt {\log n}\ge \Omega(1)\), which gives the desired lower bound \(\Delta \ge \Omega(1)/\sqrt {\log n}\).
It remains to prove Reference:maximum-of-gaussian-process. The proof considers the following family of Gaussian processes and relates their expected maxima in terms of the expected size of the matching \(M\). For \(i\in [n]\) and \(k\in \N\), let \(\super Y k_i\) be the maximum of the Gaussian process \(\{ X_j-X_i \mid j\in H^k(i)\}\), \[ \super Y k_i = \max_{j\in H^k(i)} X_j-X_i\,. \] Here, \(H^k(i)\) denotes the set of vertices that can be reached from \(i\) by at most \(k\) steps in the graph \(H\). Define the following potential function, \[ \Phi(k) = \sum_{i=1}^n \E \super Y k_i\,. \] Note that \(\Phi(k)\) allows us to lower bound \(\E \max_{i,j\in[n]}X_j-X_i\) because \(\Phi(k)/n \le \E \max_{i,j\in[n]}X_j-X_i\).
The following lemma is the key ingredient for the proof of Reference:maximum-of-gaussian-process.We remark that the proof of this lemma neither uses the triangle inequalities \eqref{eq:squared-triangle-inequality} nor the inequality \eqref{eq:well-spread}.
For every \(k\in \N\), \[ \Phi(k+1) \ge \Phi(k) + \E \card {M} - O(n)\cdot \max_{i\in [n], j\in H^{k+1}(i)} \Paren{\E (X_i-X_j)^2}^{1/2}\,. \]
This chaining lemma directly implies Reference:maximum-of-gaussian-process.
By the triangle inequality \eqref{eq:squared-triangle-inequality}, \(\E (X_i-X_j)^2 \le k \cdot \Delta\) for all \(i\in [n]\) and \(j\in H^{k}(i)\). By Reference:chaining, it follows that there exists an absolute constant \(C\ge 1\) such that for every \(k\in \N\), \(\Phi(k+1) \ge \Phi(k) + \E \card {M} - C n \cdot \sqrt{k \Delta}\,.\) For all \(k\le k_0 =(1/4C^2) \cdot (\E \card M /n)^2 / \Delta\), this inequality simplifies to \[ \Phi(k+1) \ge \Phi(k) + \tfrac 12 \E \card {M}\,. \] If we unroll this recurrence relation, we obtain the desired lower bound \[ \E \max_{i,j\in [n]} X_j -X_i \ge \Phi(k_0+1) /n \ge \tfrac 12 k_0 \Paren{\E \card M /n } = (1/8C^2) \cdot \Paren{\E \card M /n }^3 / \Delta\,. \]
The proof of Reference:chaining uses the following remarkable fact about the variance of maxima of Gaussian processes: The variance of the maximum of a collection of Gaussian variables is bounded by the maximum variance of a variable in the collection.In contrast, the expectation of the maximum typically depends also on the number of variables in the collection.
Let \(Z=(Z_1,\ldots,Z_t)\) be centered Gaussian variables. Then, the variance of the maximum of \(Z_1,\ldots,Z_t\) is bounded by \[ {\mathbb V}\Bracket{ \max\{ Z_1,\ldots,Z_t\}} \le O(1)\cdot \max\{ {\mathbb V}[Z_1],\ldots,{\mathbb V}[Z_t]\}\,. \]
The proof of this inequality also shows Gaussian-like concentration around the mean. In that form it is sometimes called Borell’s inequality and follows from Gaussian concentration inequalities for Lipschitz functions (Sudakov and Cirel\('\)son 1974; Borell 1975).See this book chapter by Pollard for different proofs of this concentration inequality.
The starting point of the proof is the following inequality among random variables for all \((i,j)\in E(H)\), \[ \super Y {k+1}_i \ge \super Y k_j + X_j -X_i\,. \] The inequality holds because \(H^{k+1}(i)\supseteq H^{k}(j)\). Hence, if \(\super Y k_j = X_h - X_j\) for some \(h\in H^{k}(j)\), then \(\super Y {k+1}_i\ge X_h - X_i = \super Y k_j + X_j -X_i\).
Let \(N\subseteq [n]\times [n]\) be an arbitrary matching of the vertices not in matching \(M\). Since matching edges \((i,j)\in M\) satisfy \(X_j-X_i \ge 2\), we can derive the following set of \(n/2\) inequalities, \[ \forall (i,j)\in M.\ \super Y {k+1}_i \ge \super Y k_j + 2 \text{ and } \forall (i,j)\in N.\ \tfrac 12 \super Y {k+1}_i + \tfrac 12 \super Y {k+1}_j \ge \tfrac 12 \super Y k_i + \tfrac 12 \super Y k_j\,. \] If we sum up these \(n/2\) inequalities, we obtain the inequality \[ \label{eq:sum-of-inequalities} \sum_{i=1}^n \super Y {k+1}_i \cdot L_i \ge \sum_{j=1}^n \super Y {k}_j\cdot R_j + 2\card{M}\,. \] Here, \(L_i=1\) if \(i\) has an outgoing edge in \(M\), \(L_i=0\) if \(i\) has an incoming edge in \(M\), and \(L_i=1/2\) if \(i\) is not matched in \(M\). Similarly, \(R_j=1\) if \(j\) has an incoming edge in \(M\), \(R_j=0\) if \(j\) has an outgoing edge in \(M\), and \(R_j=1/2\) if \(j\) is not matched in \(M\). Since every vertex is equally likely to have an incoming edge and an outgoing edge in \(M\) (see discussion after the description of the algorithm), it follows that \(\E L_i = \E R_j = 1/2\) for all \(i,j\in [n]\). Using a general bound on the variance of maxima of Gaussian processes,Here, we use the general inequality \(\Abs{\E XY - \E X \cdot \E Y}\le \sqrt{{\mathbb V}X \cdot {\mathbb V}Y}\), which we leave as an exercise to the reader. \[ \Abs{\E \super Y {k+1}_i \cdot L_i - \E \super Y {k+1}_i \cdot \E L_i }\le \sqrt{{\mathbb V}\Bracket{\super Y {k+1}_i }\cdot {\mathbb V}[L_i]} \le O(1) \cdot \max_{j\in H^{k+1}(i)} \Paren{\E (X_i-X_j)^2}^{1/2}\,. \] Similarly, \[ \Abs{\E \super Y {k}_j \cdot R_j - \E \super Y {k}_j \cdot \E R_j} \le \sqrt{{\mathbb V}\Bracket{\super Y {k}_j }\cdot {\mathbb V}[ R_j]} \le O(1) \cdot \max_{i\in H^{k}(j)} \Paren{\E (X_i-X_j)^2}^{1/2}\,. \] By taking the expectation of inequality \eqref{eq:sum-of-inequalities} and applying the above deviation bounds, it follows as desired that \[ \sum_{i=1}^n \E \super Y {k+1}_i \ge \sum_{j=1}^n \E \super Y {k}_j + 4\card{M} - O(n)\cdot \max_{i\in [n],j\in H^{k+1}(i)} \Paren{\E (X_i-X_j)^2}^{1/2}\,. \]
Reduction to the well-spread case
We describe now how to prove Reference:arora-rao-vazirani-approximation-min-expansion in case inequality \eqref{eq:well-spread} is not satisfied.
Recall the distance \(d(i,j)=\E_{\mu(x)}(x_i-x_j)^2\). Let \(\delta>0\) be the average distance \[ \delta=\tfrac 1 {n^2} \sum_{i,j\in[n]} d(i,j)\,. \label{eq:average-distance} \]
The following lemma shows that if there exists a cluster of radius significantly smaller than \(\delta\) that contains a constant fraction of vertices, then the condition of Reference:region-growing is satisfied for \(\Delta\ge \Omega(1)\) (which means that we get a \(O(1)\)-approximation).
Suppose there exists \(i\in [n]\) such that \(A=\{j\in [n] \mid d(i,j)\le \delta/4\}\) satisfies \(\card A \ge \Omega(n)\). Then, \(\card A \cdot \sum_{j=1}^n d(j,A)\ge \Omega(1)\cdot \sum_{i,j\in[n]} d(i,j)\).
By the triangle inequality, \[ \delta \le \tfrac 1 {n^2} \cdot \sum_{i,j\in [n]} d(i,A)+d(j,A) + \delta/2 \le \delta/2 + \tfrac 1{n} \sum_{i=1}^n 2d(i,A) \,. \] Thus, \(\card{A}\sum_{j=1}^n d(j,A)\ge n\cdot \delta/4\cdot \card{A}\ge \Omega(1)\cdot \delta n^2\), which is the desired bound.
The following lemma shows that if the condition of the previous lemma is not satisfied, then after restricting to a constant fraction of the vertices there are Gaussian random variables that we can apply the structure theorem to in order to obtain sets \(A,B\subseteq[n]\) with \(\card{A},\card{B}\ge \Omega(n)\) such that \(d(i,j)\ge \Omega(1/\sqrt{\log n})\cdot \delta\) for all \(i\in A\) and \(j\in B\).
Either there exists a vertex \(i\in[n]\) that satisfies the condition of Reference:heavy-cluster or there exists a subset \(U\subseteq [n]\) with \(\card U \ge \Omega(n)\) and a centered Gaussian vector \(Y=(Y_1,\ldots,Y_n)\) such that
- \(\E Y_i^2\le 1\) for all \(i\in U\),
- \(\sum_{i,j\in U}\E (Y_i-Y_j)^2\ge 0.05\),
- there exists \(\alpha\ge \Omega(\delta)\) such every \(i,j\in U\) satisfies \(d(i,j) = \alpha \cdot \E (Y_i-Y_j)^2\).
Suppose that no vertex \(i\in [n]\) satisfies the condition of Reference:heavy-cluster. So every \(i\in [n]\) satisfies \(\card{B(i,\delta/4)}\ge 0.1n\), where \(B(i,\delta/4)\) is the set of vertices \(j\in[n]\) such that \(d(i,j)\le\delta/4\). Let \(k \in [n]\) be such that \(\sum_{i=1}^n d(i,k)\le \delta n\). Let \(U\subseteq [n]\) be the set of vertices \(i\in[n]\) such that \(d(i,k)\le 2\delta\). By Markov’s inequality, \(\card{U}\ge n/2\). Then, \[ \sum_{i,j\in U} d(i,j) \ge \sum_{i\in [n]} \delta/4 \cdot \card{U\setminus B(i,\delta/4)} \ge n \cdot \delta/4 \cdot 0.4 n= 0.1\delta n\,. \] By symmetry, we may assume \(\pE_{\mu(x)}x=\tfrac 12 \cdot \Ind\). Therefore, if we let \(X_1,\ldots,X_n\) be the Gaussian variables with the same first two moments as the pseudo-distribution \(\mu\). Then, the variables \(Y_1,\ldots,Y_n\) with \(Y_i=(X_i-X_k)/\sqrt{2\delta}\) satisfy \(\E Y_i^2 \le 1\) for all \(i\in [n]\), \(\sum_{i,j\in U}\E (Y_i-Y_j)^2\ge 0.05\) and \(\E (Y_i-Y_j)^2 = d(i,j)/2\delta\).
References
Arora, Sanjeev, Satish Rao, and Umesh V. Vazirani. 2004. “Expander Flows, Geometric Embeddings and Graph Partitioning.” In STOC, 222–31. ACM.
Borell, Christer. 1975. “The Brunn-Minkowski Inequality in Gauss Space.” Invent. Math. 30 (2): 207–16.
Leighton, Frank Thomson, and Satish Rao. 1988. “An Approximate Max-Flow Min-Cut Theorem for Uniform Multicommodity Flow Problems with Applications to Approximation Algorithms.” In FOCS, 422–31. IEEE Computer Society.
Rothvoss, Thomas. 2016. “Lecture Notes on the ARV Algorithm for Sparsest Cut.” CoRR abs/1607.00854.
Sudakov, V. N., and B. S. Cirel\('\)son. 1974. “Extremal Properties of Half-Spaces for Spherically Invariant Measures.” Zap. Naučn. Sem. Leningrad. Otdel. Mat. Inst. Steklov. (LOMI) 41: 14–24, 165.